This article introduces the in silico drug discovery technique called Fragment-based Drug Design (FBDD). It explains how to use the FBDD software ACFIS 2.0 to discover drugs for Parkinson’s disease on your own computer and also provides specific compounds as examples. By learning about it, you can understand the latest drug discovery technology, ACFIS 2.0, and experience drug discovery on your own computer. It would be valuable information for those interested in pharmaceutical development and pharmacy. Please give it a try!
macOS Ventura(13.2.1)
What is Fragment-based in silico drug design?
Fragment-Based Drug Design (FBDD) is an in silico drug discovery approach that employs computer-based techniques to design and develop new drugs. This method utilizes small molecular fragments or fragments derived from existing ligands to construct and optimize drug molecules. FBDD offers an efficient and cost-effective approach to designing novel drug candidates by analyzing complex molecular interactions and leveraging known drugs. Moreover, FBDD reduces the burden of experimental screening and chemical synthesis, improving drug discovery speed and efficiency.
What is ACFIS 2.0?
ACFIS (Auto Core Fragment in silico Screening) server is a powerful online tool for identifying potential drug leads through FBDD. However, accurately predicting the binding modes and affinities of protein-fragment interactions remains a challenge due to weak binding affinities. To address this, ACFIS 2.0 was developed, incorporating a dynamic fragment growth strategy to account for protein flexibility. Key improvements in ACFIS 2.0 include: (i) enhanced accuracy in identifying hit compounds (from 75.4% to 88.5% using the same test set), (ii) improved rationalization of protein-fragment binding modes, (iii) expanded fragment library for increased structural diversity, and (iv) additional comprehensive functionalities for predicting molecular properties. ACFIS 2.0 has successfully led to the discovery of drug lead compounds for Parkinson’s disease, cancer, and severe depressive symptoms.
This article will demonstrate the usage of ACFIS 2.0 through the example of Parkinson’s disease drugs as described in the research paper.
ACFIS 2.0: an improved web-server for fragment-based drug discovery via a dynamic screening strategy
Protein Input and Library Selection
Let’s dive into FBDD using ACFIS! First, visit the ACFIS website.
Then, follow these steps:
- Choose the desired protein from the Protein Data Bank (PDB). For this demonstration, we will select monoamine oxidase-B (MAO-B) (PDB: 2v5z), which is a target for Parkinson’s disease.
- Select the library to be used. In this case, we will use the Approved Drug library.
- Fill in the remaining details. Setting a password will ensure that only you can access the results.
- Click “Submit.”
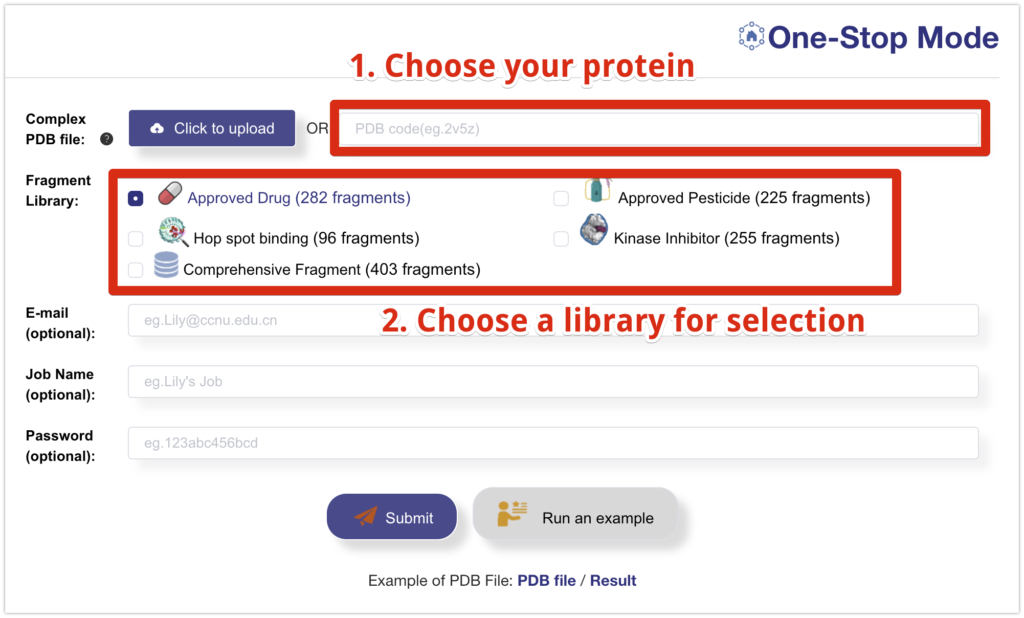
5. Select the ligands to be used for FBDD and click Submit for Screening. In this case, we will use the compounds on the right side.
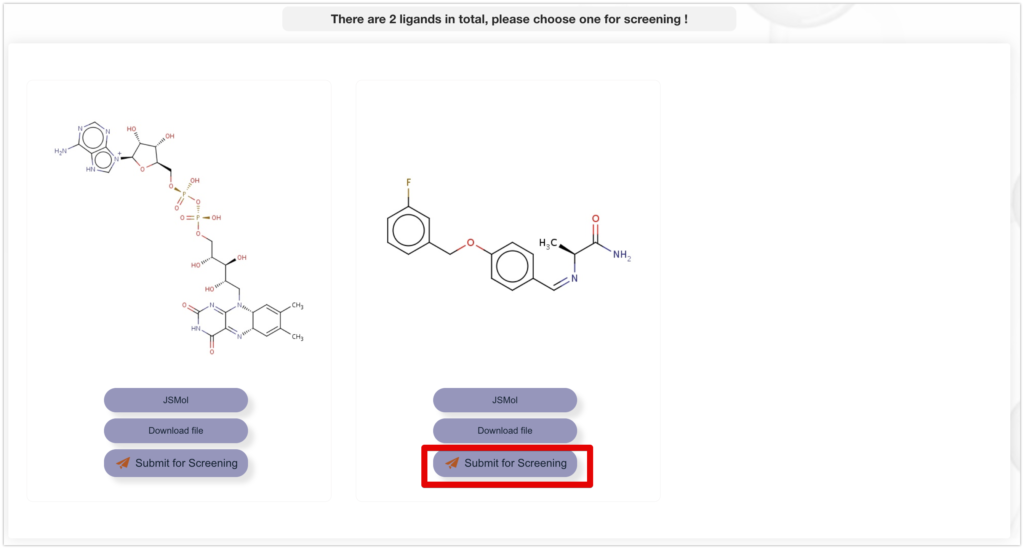
That’s all for the setup. FBDD will be performed automatically, and the results will be outputted after some time, taking approximately 1-6 hours.
Here, we will perform FBDD targeting monoamine oxidase-B (MAO-B) (PDB: 2v5z), which is a target for Parkinson’s disease. We will use the Approved Drug library. (The original paper used the Comprehensive Fragment library.)
Parkinson’s disease is a condition where there is a deficiency of dopamine in the brain, resulting in difficulty in movement. Dopamine is produced in the substantia nigra in the brain, but in Parkinson’s disease, the accumulation of Lewy bodies in the substantia nigra leads to a decrease in dopamine-producing neurons and a reduction in dopamine production. The symptoms of Parkinson’s disease can be classified into motor symptoms and non-motor symptoms. Motor symptoms include tremors in the hands, legs, chin, etc., stiffness of the muscles in the limbs, slowed movements, and impaired balance. Non-motor symptoms include constipation, frequent urination, dizziness, depressed mood, anxiety, hallucinations, loss of smell, insomnia, daytime sleepiness, forgetfulness, and poor attention. Treatment options include medication therapy and rehabilitation. Medications used in the treatment include MAO-B inhibitors, which inhibit the breakdown of dopamine, and dopamine agonists.
MAO-B is one type of monoamine oxidase, an enzyme that breaks down dopamine, serotonin, and norepinephrine. In Parkinson’s disease, MAO-B inhibitors are used to inhibit the function of MAO-B, thereby increasing dopamine levels in the brain and improving motor symptoms.
ACFIS 2.0 Workflow
Although there isn’t much for you to do here, let’s explain the mechanism of ACFIS 2.0.
The overall workflow of FBDD using ACFIS 2.0 can be divided into two modules and four steps. The starting module is called CORE GEN, which is designed to generate core fragments from the given ligand structures. This module consists of two steps: fragment decomposition and identification of core fragments. The purpose of the second module, CAND GEN, is to derive candidate compounds from core fragments. The CAND GEN module consists of two sequential steps: dynamic fragment growth based on the identified core fragments in the first module and molecular property evaluation for candidate selection. The core processes of the ACFIS 2.0 pipeline are organized based on the previously developed Pharmacophoric Fragment Virtual Screening (PFVS) method. Details of the four steps are described in Supplementary Text S1.
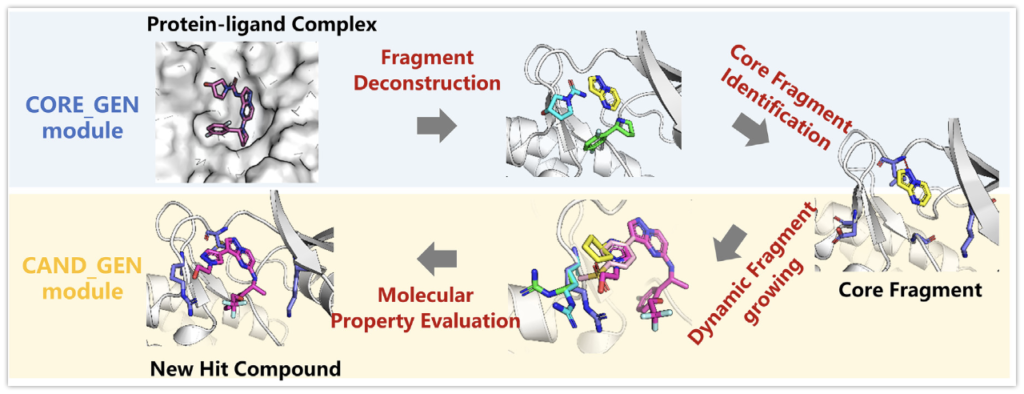
Results
If the status in Jobs is finished, the analysis is completed. Select the analysis you performed based on the JOB ID and go to the analysis page. The protein-ligand complex can be viewed in 3D on the screen. The list on the left represents the ligands, with the initially inputted ligand at the top, followed by the ligands arranged in descending order of binding affinity. Physical properties of the binding ligands are also summarized.
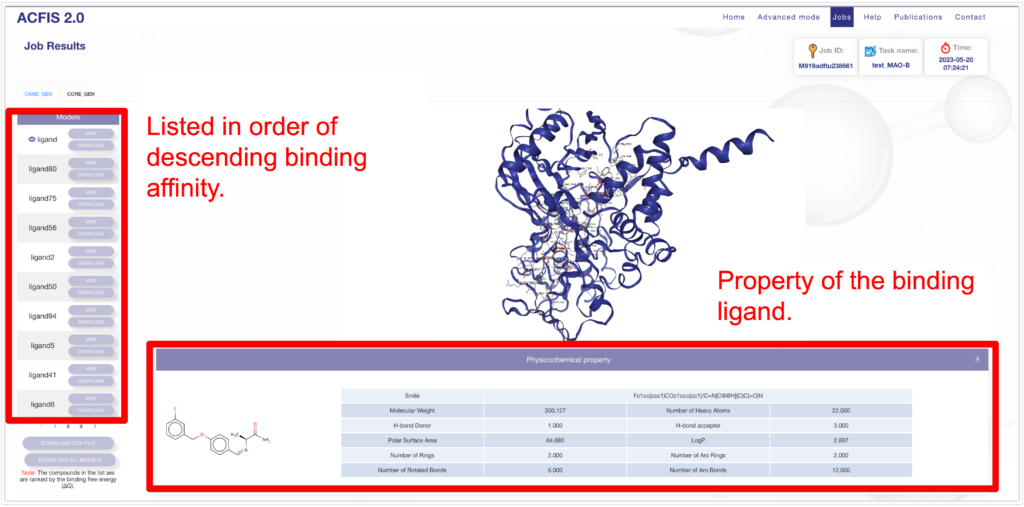
As you scroll down, detailed data is displayed.
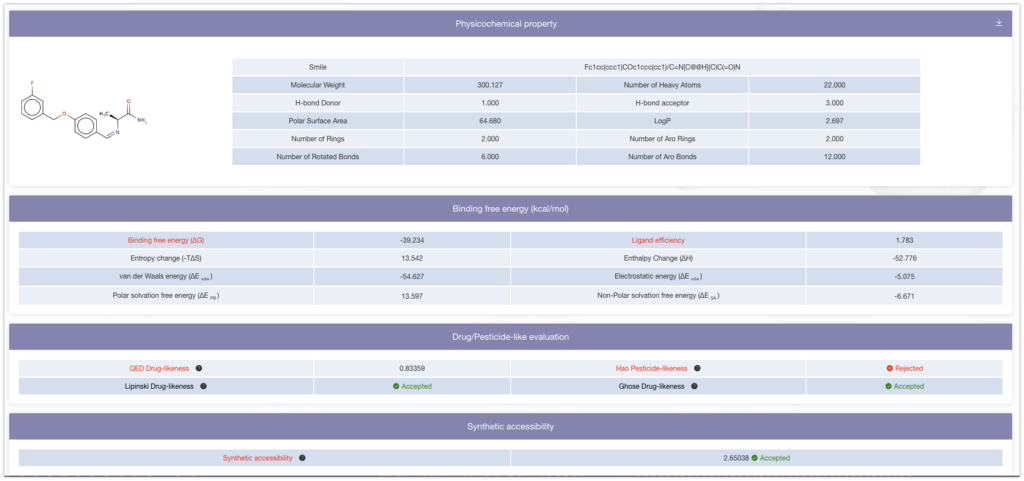
- Molecular Weight: Indicates the mass of the compound. Higher molecular weight indicates larger compounds, which may result in reduced biological availability as drugs.
- Number of Heavy Atoms: : Indicates the number of non-hydrogen atoms in the compound. A higher number of heavy atoms indicates larger compounds.
- H-bond Donor & H-bond acceptor: Indicates the number of hydrogen bond donors and acceptors in the compound. A higher number suggests that the compound may have stronger interactions with other molecules.
- Polar Surface Area: Indicates the surface area of the polar portion of the molecule. A larger polar surface area suggests higher water solubility but may indicate lower ability to pass through cell membranes.
- LogP: A parameter that indicates the compound’s hydrophobicity, represented by the logarithm of the partition coefficient between water and octanol. Higher LogP values suggest higher hydrophobicity and potentially better ability to pass through cell membranes.
- Number of Rings & Number of Aro Rings: Indicates the number of ring structures in the compound. A higher number suggests greater complexity in the compound.
- Number of Rotated Bonds & Number of Aro Bonds: Indicates the number of rotatable bonds and aromatic bonds in the compound. A higher number suggests greater complexity in the compound.
- Binding free energy (ΔG): Represents the energy change when the ligand binds to the target. A lower ΔG indicates a stronger binding between the ligand and the target.
- Ligand efficiency: Normalized binding free energy based on the number of heavy atoms in the ligand. Higher ligand efficiency suggests strong binding of the ligand to the target relative to its size.。
- Entropy change (-TΔS), Enthalpy Change (ΔH), van der Waals energy (ΔE vdw ), Electrostatic energy (ΔE ele ), Polar solvation free energy (ΔE PB ), Non-Polar solvation free energy (ΔE SA ): These parameters provide detailed information about the energy changes when the ligand binds to the target. Larger values indicate stronger binding between the ligand and the target. These parameters represent the natural state of binding, and larger values suggest greater stability in the ligand-target binding.
- Drug/Pesticide-like evaluation: Higher scores indicate a higher likelihood that the compound possesses characteristics of a drug or pesticide. These evaluations reflect the potential for the compound to exhibit biological activity and safety towards the human body and the environment.
- Synthetic accessibility: Indicates the ease of synthesizing the compound in the laboratory. Low synthetic accessibility suggests difficulties in synthesizing the compound. This is an important consideration in the development of new drug candidates, as compounds with high synthetic accessibility are more likely to be easily developed and manufactured.
Based on the results, we were able to discover a ligand (Binding Free Energy: ΔG = -55.931) that has a higher binding affinity than the ligand you initially introduced (Binding Free Energy: ΔG = -39.23).
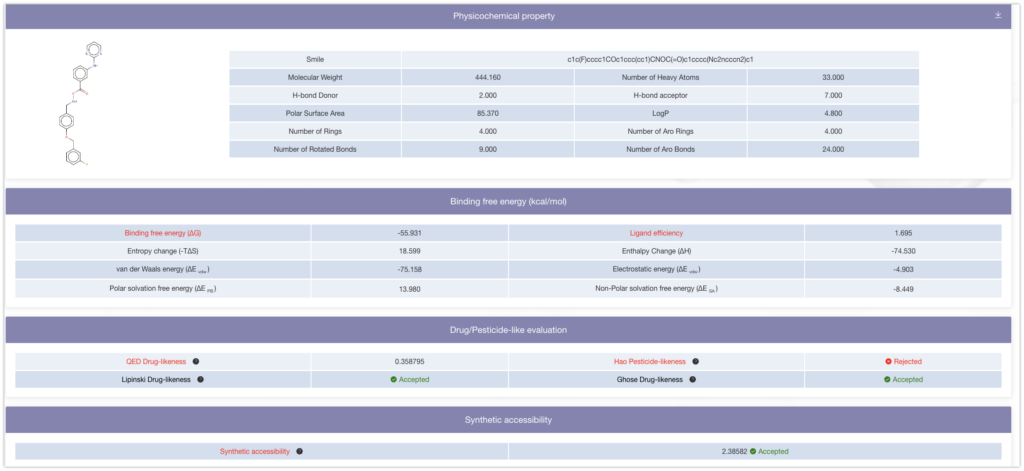
Final Thoughts
How was your experience? Being able to easily discover compounds with potential high activity through browser operations is quite appealing! Changing the library will yield different output compounds, so feel free to experiment with various options. Please give it a try and make use of it to improve the activity of compounds!
Original Paper:
ACFIS 2.0: an improved web-server for fragment-based drug discovery via a dynamic screening strategy